Researchers at The George Washington University are developing innovative analytics for power grid online surveillance (event detection and classification with minimum latency) and real-time situational awareness. The novel analytics can be deployed as a stand-alone next-generation smart sensor or can be embedded within the existing phasor measurement units (PMUs) and intelligent electronic devices (IEDs), and features (i) event detection and classification in power grids of different sizes and characteristics, (ii) accurate and fast detection of almost all types of events that can happen in power grids (different types of faults—single-line-to-ground, 3-phase, double-line-to-ground, etc.—, surges, voltage sags, power swings, load changes, topology changes, etc.). This approach revolutionizes the existing measurement and monitoring paradigms (centralized) in power grids to a high-fidelity distributed setting for measurement (sensing) and decision making (actuating) setting.
According to ‘’QYresearch”, the accumulated global market size for PMU devices and techniques is expected to reach $816 Million by the end of 2025. The existing technology, i.e., PMUs located in power substations, measure the voltage and current phasors continuously and report them to the control centers through communication channels. The control center applications then utilize such data to analyze and monitor the power grid. This current practice heavily relies on reliable and secure communication gateways: if the communication channels are lost (due to failures or cyber-attacks) or have delays, then the control center analytics and application trustworthiness will be compromised or will be done with latencies.
Professor Payman Dehghanian and his students have invented a paradigm shift for event detection and classification in power grids by developing advanced analytics closer to where the data is generated and measurements are captured, i.e., at the substations and embedded within the PMU devices. The proposed analytics facilitate development and deployment of the next-generation smart sensors in power grids and relaxes the need to transmit high-volumes of data from power grid into central control centers for decision making, but to embed additional levels of smartness for distributed decision making. This solution makes it possible to achieve an online surveillance and monitoring of the grid in real-time and with minimum latency, as it is no longer susceptible to communication failures and vulnerabilities.
The inputs to the proposed analytics are the power grid waveforms, which are analyzed for event detection and classification outputs. This technology, different from the stat-of-the-art, does not rely on synchrophasor estimates (PMU outputs), but only works on power waveforms that are captured at the measurement points (substations). Hence, the proposed technology is not vulnerable to PMU errors and latencies, making it possible for real-time online monitoring of the grid.
To be more specific, the first module is a pseudo continuous quadrature wavelet transform (PCQ-WT) algorithm using a modified Gabor wavelet transform, which generates the featured-scalograms for pattern recognition and feature extractions (i.e., to capture the waveform fingerprints); and the second module is a convolutional neural network (CNN) machine learning technology that classifies the events based on the extracted features in the scalograms. The experiments demonstrate that the proposed framework achieves a promising classification accuracy on multiple types of prevailing events in power grids, through which an enhanced grid-scale situational awareness in real-time can be realized. The proposed concept is proven, and currently a software prototype is developed that works as expected.
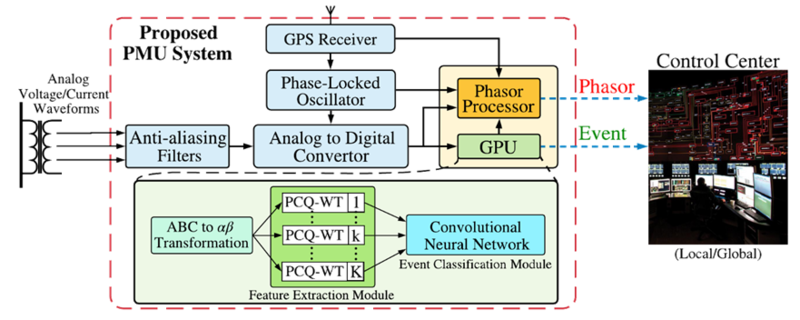
Applications:
1. Power grid online surveillance in both transmission and distribution systems
2. Fast and accurate post-event analysis;
3. Stability Monitoring;
4. Fault Analysis: Fault Detection, Fault Type Classification, Fault Location
5. Advanced Control Schemes: Wide Area Protection and Control.
Advantages:
1. Resistive to timing synchronization failures, communication losses (failures or attacks), data transmission delays
2. Self-reliant on original power waveforms and no vulnerability to PMU errors and latencies
3. High accuracy rate of 94.7% and a faster reporting speed at a rate higher than 60 samples per second than standard PMUs.
4. Real-time situational awareness with online distributed event detection and classification